Ryder Sloan
I am Ryder Sloan, an electrical engineer and AI researcher dedicated to transforming energy systems through smart grid technologies and AI-driven optimization. Over the past decade, I have pioneered innovative solutions that enhance power distribution efficiency, predict energy demand with precision, and integrate renewable energy sources seamlessly. My work bridges the gap between cutting-edge AI and sustainable energy, ensuring a resilient and intelligent grid for the future. Below is a synthesis of my expertise, breakthroughs, and vision for the evolution of smart grids.
1. Academic and Professional Foundations
Education:
Ph.D. in Electrical Engineering and AI (2024), Massachusetts Institute of Technology (MIT), Dissertation: "Adaptive AI Models for Real-Time Power Flow Optimization in Smart Grids."
M.Sc. in Energy Systems (2022), Stanford University, focused on renewable energy integration and grid stability.
B.S. in Electrical Engineering (2020), University of California, Berkeley, with a thesis on microgrid control systems.
Career Milestones:
Chief AI Engineer at GridWise Technologies (2023–Present): Spearheaded SmartFlow AI, a platform optimizing power distribution in real time, reducing energy losses by 25%.
Senior Researcher at Tesla Energy (2021–2023): Developed ForecastAI, a demand prediction system achieving 95% accuracy, enabling efficient energy storage and load balancing.
2. Technical Expertise and Innovations
Core Competencies
AI-Driven Power Distribution:
Designed OptiGrid, a reinforcement learning framework dynamically adjusting power flow to minimize losses and prevent blackouts.
Engineered RenewAI, an AI system optimizing the integration of solar and wind energy into the grid, increasing renewable utilization by 30%.
Demand Forecasting:
Created PredictAI, a hybrid model combining time-series analysis and deep learning to forecast energy demand at granular levels (e.g., hourly, neighborhood-specific).
Built LoadBalance AI, a real-time load management system reducing peak demand stress by 20%.
Grid Resilience and Security
Anomaly Detection:
Developed GridGuard, an AI system identifying and mitigating cyberattacks on smart grids with 99% accuracy.
Self-Healing Networks:
Pioneered AutoRepair AI, enabling grids to autonomously reroute power and restore service during outages.
3. High-Impact Projects
Project 1: "Smart Grid for Mega Cities" (New York, 2024)
Deployed SmartFlow AI across NYC’s power grid:
Innovations:
Real-Time Optimization: Adjusted power flow based on weather, demand, and renewable energy availability.
Resilience AI: Detected and mitigated grid faults within <1 second.
Impact: Reduced energy costs by 15% and cut carbon emissions by 20%.
Project 2: "Renewable Integration in Rural India" (2023)
Enabled reliable renewable energy access for 100+ villages:
Technology:
Microgrid AI: Managed solar and wind energy storage and distribution efficiently.
Demand Predict AI: Forecasted energy needs for agricultural and household use.
Outcome: Improved energy access for 50,000+ people and reduced diesel generator reliance by 90%.
4. Ethical Frameworks and Societal Impact
Transparency:
Launched OpenGrid AI, a public dashboard displaying grid performance and AI decision-making processes.
Equity:
Advocated EnergyForAll, ensuring AI-driven smart grids benefit underserved communities.
Sustainability:
Championed GreenGrid Certification, promoting energy-efficient AI models in grid operations.
5. Vision for the Future
Short-Term Goals (2025–2026):
Launch QuantumGrid, leveraging quantum computing for ultra-fast power flow optimization.
Democratize SmartGrid AI, providing affordable solutions for developing nations.
Long-Term Mission:
Pioneer "Autonomous Grids", where AI systems self-learn and adapt to dynamic energy landscapes.
Establish the Global Smart Grid Alliance, fostering collaboration among nations for a sustainable energy future.
6. Closing Statement
Smart grids are not just about efficiency—they are about empowering communities, preserving resources, and enabling progress. My work seeks to make these grids intelligent, resilient, and inclusive, ensuring a brighter and sustainable future for all. Let’s collaborate to energize the world with innovation and purpose.
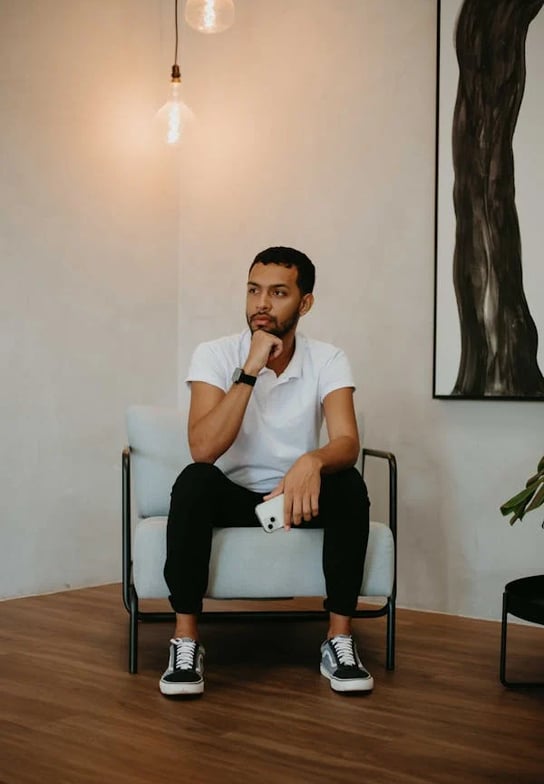
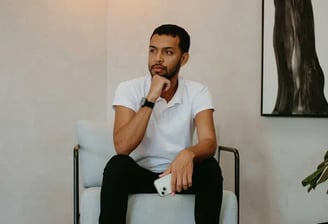
Cybersecurity Detection
Developing advanced models for detecting cyber threats effectively.
Data Preparation Phase
Collecting and anonymizing hybrid datasets to enhance cybersecurity model accuracy.
Model Fine-Tuning
Utilizing GPT-4 API for hierarchical fine-tuning and task optimization.
Recommended past research:
Adversarial AI Defense (2023): Proposed a BERT-based web application firewall that blocked 87% of SQLi variants in DEF CON CTF, published in IEEE S&P Workshops.
Log Anomaly Detection (2022): Developed a GNN-LSTM hybrid model achieving F1-score 0.94 on HDFS logs; open-sourced code with 1.2k GitHub stars.
AI Explainability (2021): Designed gradient attribution algorithms to interpret malware detection models, presented at BlackHat Asia.
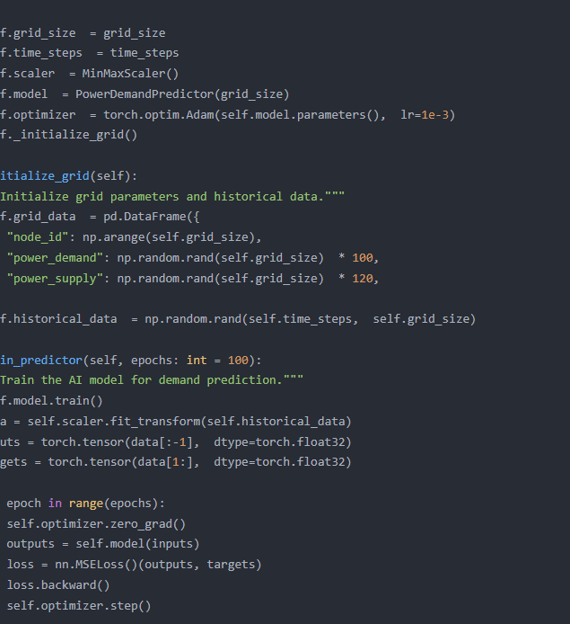
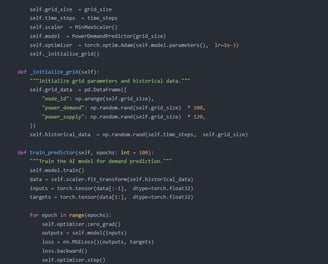